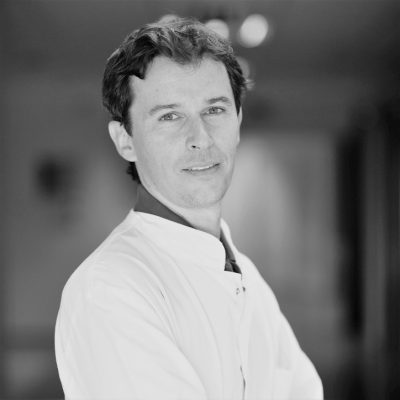
Olivier HUMBERT
PET/CT imaging and Artificial Intelligence for precision Oncology
Main interests
- PET/CT imaging
- Imaging of tumor response to immunotherapy
- Predictive Artificial Intelligence in oncology
- Federated Learning
Scientific Questions
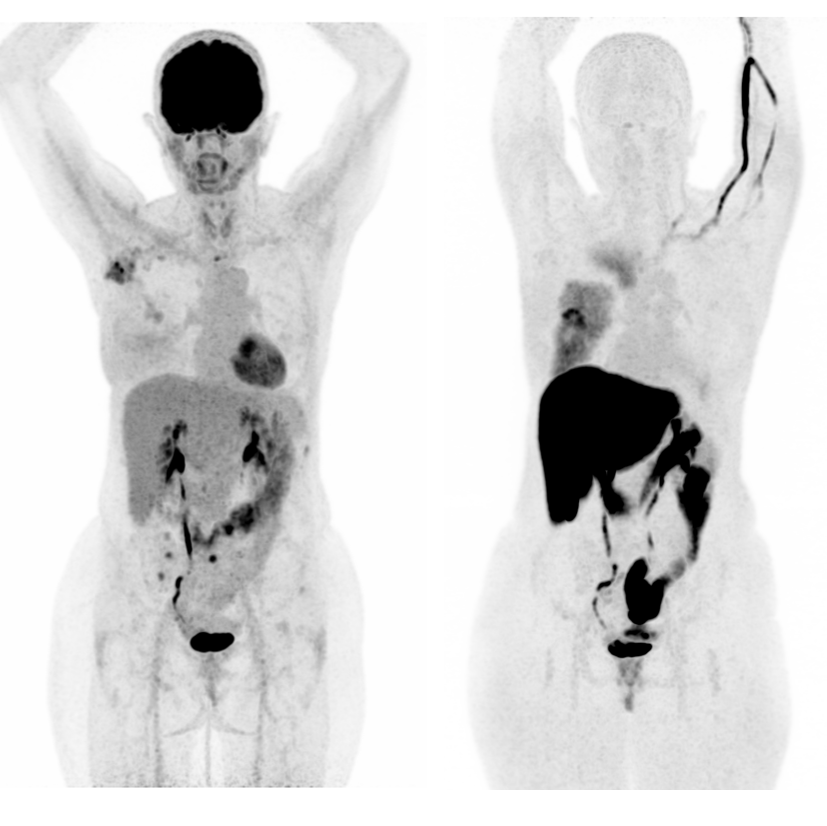
Therapeutic advances have recently improved the outcome of patients with metastatic cancer. In non-small cell lung cancer, immune checkpoint inhibitors (i.e. immunotherapy) are a major therapeutic breakthrough, while the systematic combination of CDK4/6 inhibitors with hormonotherapy is the new standard for patients with metastatic ER+ breast cancer. However, therapeutic resistance remains a major challenge, and new predictive biomarkers of treatment efficacy are needed to better guide the therapeutic strategy. Monitoring tumor response using clinical imaging, such as CT or FDG-PET, has also become very challenging in the area of immunotherapy due to atypical evolutionary patterns such as pseudo-progression and dissociated response. New response criteria are needed for the follow-up of treated patients.
Our Strategy
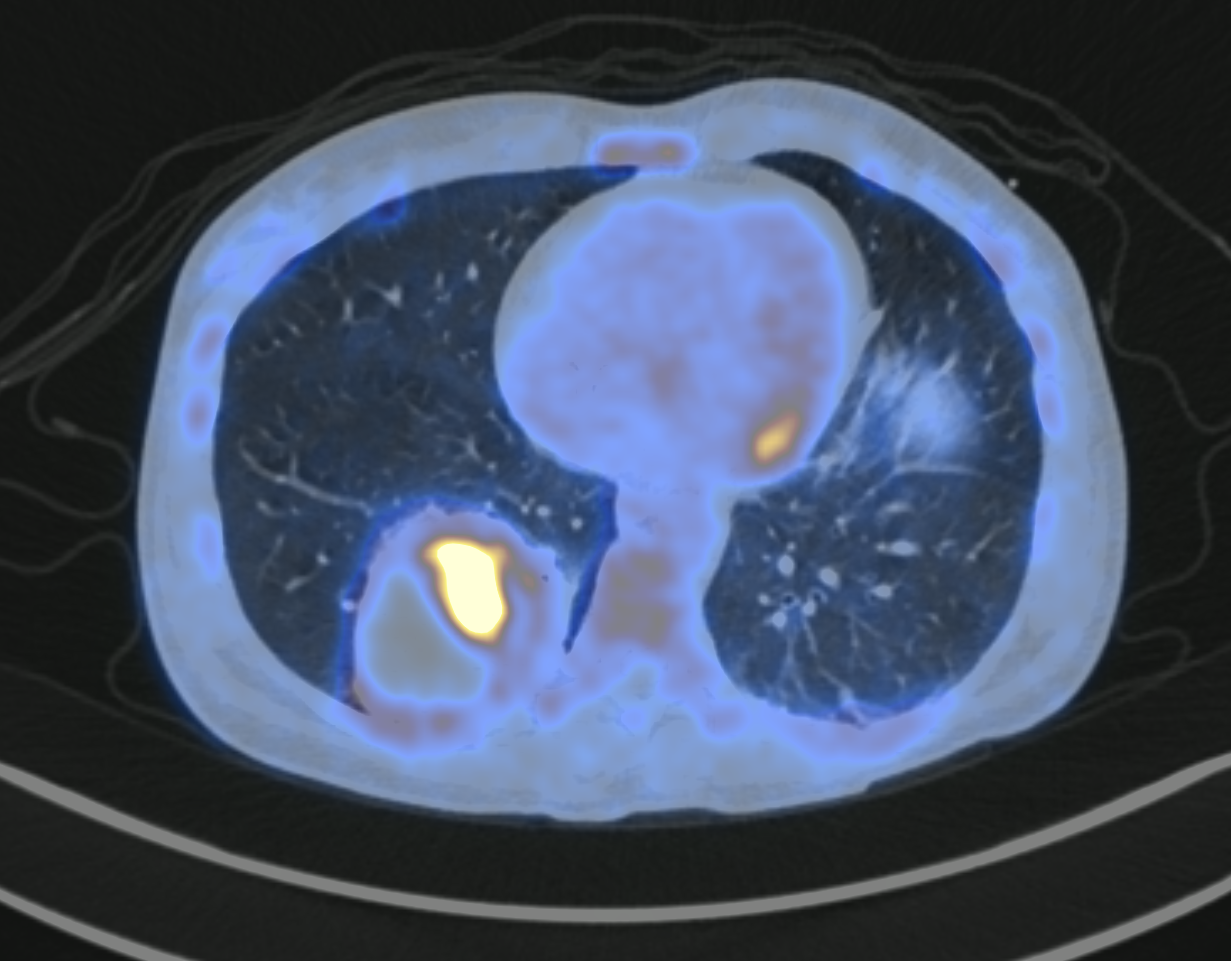
Beyond tumor biology, molecular whole-body imaging, such as 18FDG PET-CT, offers significant potential in terms of non-invasive biomarkers for predicting and monitoring tumor response to treatment. In the metastatic setting, PET/CT enables in vivo analysis of all metastatic lesions in a single patient, taking into account tumor heterogeneity between metastases and the patient’s immune background. In addition to the discovery of individual imaging biomarkers, radiomics is a promising approach to better predict a patient's response to a particular treatment. Radiomics involves the extraction of several dozens of quantitative indexes extracted from the medical image and their co-analysis by Machine Learning algorithms. Convolutional Neural Networks (CNNs), based on Deep Learning, are also statistical approaches with impressive performance for tasks linked to computer-aided diagnosis, such as image classification, lesion segmentation or tracking. Deep learning algorithms can identify subtle predictive patterns hidden in medical images. However, to be generalizable, the training of Deep Learning models requires large multicentric medical dataset, which are currently complex to obtain due to the sensitive nature of medical data.
Research Aims
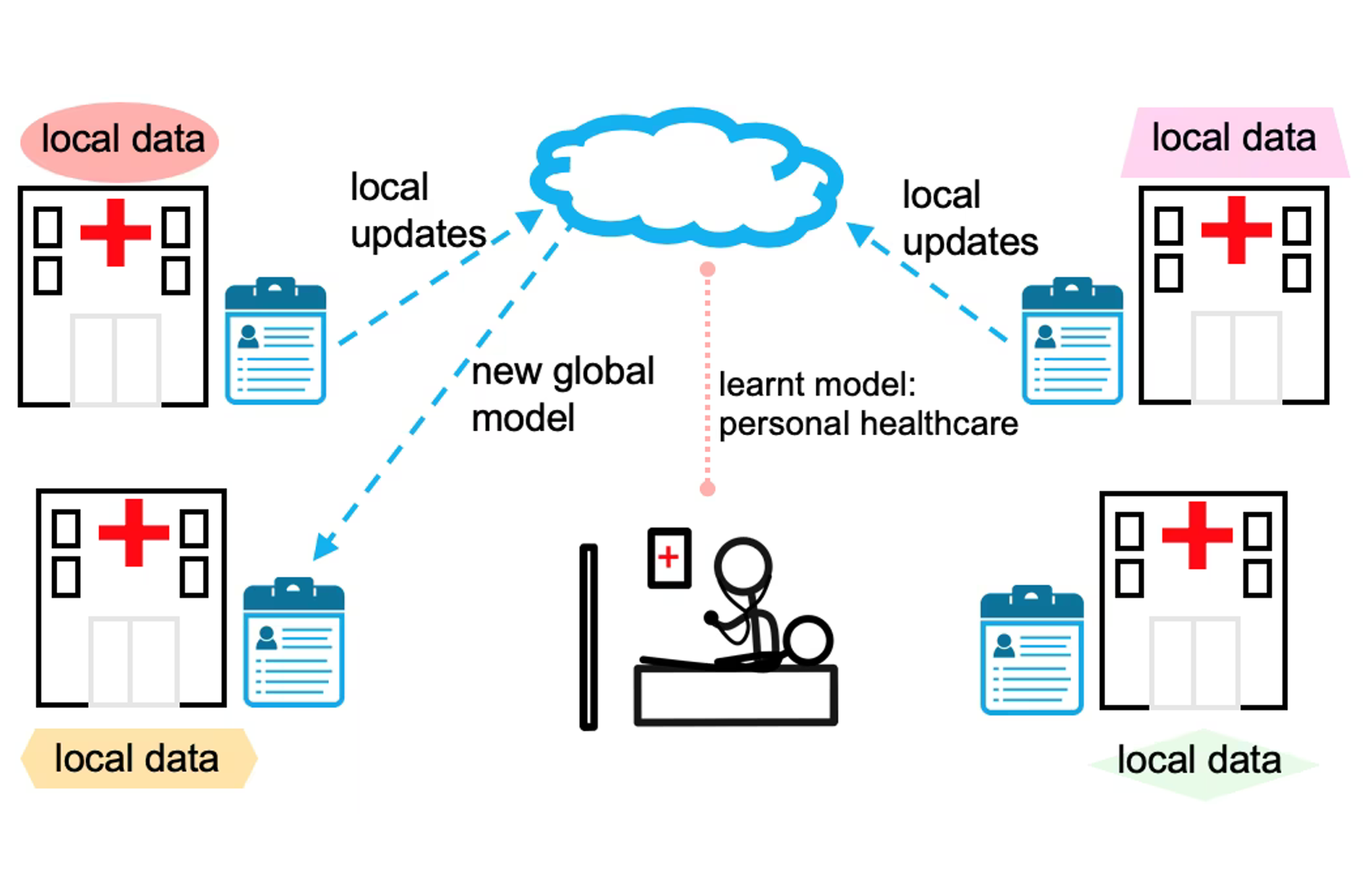
The identification of PET-CT biomarkers predictive of the lung cancer response to immunotherapy and of breast cancer response to hormone therapy. Beyond the discovery of standard imaging biomarkers, the team also aims to exploit the full potential of radiomics and deep learning to maximize the extraction of the prognostic information from PET/CT images. Working closely with research teams in applied mathematics, we aim to offer “AI-based precision oncology” and address the challenge of bringing AI models from the lab to the clinic. Among the main issues for development of AI algorithms is the availability of large, curated and multicentric training medical data, stored in a unique and centralized repository. To tackle this problem, work is being carried out on so-called federated learning technologies (PRT-K 2022 funding). The FEDERATED-PET project aims at establishing the first French Federated Learning (FL) initiative for the collaborative Deep Learning analysis of 3D PET/CT images and clinical data, leveraging on state-of-the-art FL platforms (FedBio-Med, INRIA) and an important network of hospitals. FL provides will create a unique opportunity to collaboratively learn a shared model across multiple hospitals, solving the crucial problem of medical data sharing and exchange.
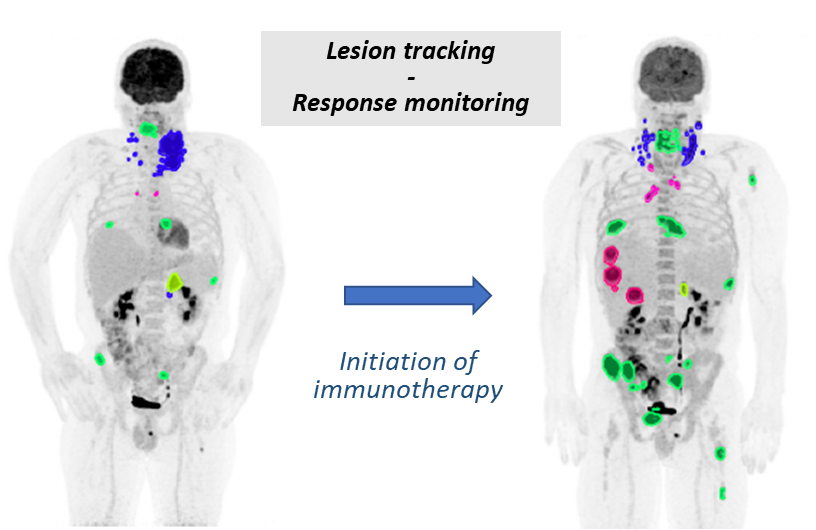
Improving the monitoring of tumor response to immunotherapy using Artificial Intelligence. This project is a collaboration between the Université Côte d'Azur, the Antoine Lacassagne Cancer Center and EuraNova. It aims to develop a deep learning algorithm for the automatic analysis of longitudinal PET/CT images, enabling 1) the automatic detection of multiple metastatic lesions on a single PET scan 2) the temporal tracking of these lesions between two consecutive PET scans. This innovative tool will 1) help physicians to analyze and classify tumor response (saving time / reducing errors) 2) automatically quantify changes in metabolic indices of lesions after the start of treatment, providing a new generation of AI-based tumor response criteria. Focusing on lung cancer patients treated with immune checkpoint inhibitors, it will enable better discrimination between patients who respond well or poorly to immune checkpoint inhibitors, taking into account atypical evolutive patterns such as pseudo-progression. The clinical benefit will be to optimize the therapeutic strategy for a more personalized medicine.
Researchers
CHARDIN David - +33 R
PreDocs
SCHMUTZ Hugo - +33 R
COMTE Victor - +33 R
Students
MASSE Mathilde - +33 R
Recent publications
- Tricarico, P, Chardin, D, Martin, N, Contu, S, Hugonnet, F, Otto, J et al.. Total metabolic tumor volume on 18F-FDG PET/CT is a game-changer for patients with metastatic lung cancer treated with immunotherapy. J Immunother Cancer. 2024;12 (4):. doi: 10.1136/jitc-2023-007628. PubMed PMID:38649279 PubMed Central PMC11043703.
- Bailleux, C, Chardin, D, Guigonis, JM, Ferrero, JM, Chateau, Y, Humbert, O et al.. Survival analysis of patient groups defined by unsupervised machine learning clustering methods based on patient metabolomic data. Comput Struct Biotechnol J. 2023;21 :5136-5143. doi: 10.1016/j.csbj.2023.10.033. PubMed PMID:37920813 PubMed Central PMC10618114.
- Bailleux, C, Chardin, D, Gal, J, Guigonis, JM, Lindenthal, S, Graslin, F et al.. Metabolomic Signatures of Scarff-Bloom-Richardson (SBR) Grade in Non-Metastatic Breast Cancer. Cancers (Basel). 2023;15 (7):. doi: 10.3390/cancers15071941. PubMed PMID:37046602 PubMed Central PMC10093598.
- Lévi-Strauss, T, Tortorici, B, Lopez, O, Viau, P, Ouizeman, DJ, Schall, B et al.. Radiomics, a Promising New Discipline: Example of Hepatocellular Carcinoma. Diagnostics (Basel). 2023;13 (7):. doi: 10.3390/diagnostics13071303. PubMed PMID:37046521 PubMed Central PMC10093101.
- Chardin, D, Gille, C, Pourcher, T, Humbert, O, Barlaud, M. Learning a confidence score and the latent space of a new supervised autoencoder for diagnosis and prognosis in clinical metabolomic studies. BMC Bioinformatics. 2022;23 (1):361. doi: 10.1186/s12859-022-04900-x. PubMed PMID:36050631 PubMed Central PMC9434875.
- Khatir, W, Humbert, O, Benzaquen, J, Bontoux, C, Neels, J, Berland, L et al.. Identification of a circulating immunological signature predictive of response to immune checkpoint inhibitors in patients with advanced non-small cell lung cancer. Clin Transl Med. 2022;12 (8):e1018. doi: 10.1002/ctm2.1018. PubMed PMID:35994416 PubMed Central PMC9394752.
- Comte, V, Schmutz, H, Chardin, D, Orlhac, F, Darcourt, J, Humbert, O et al.. Development and validation of a radiomic model for the diagnosis of dopaminergic denervation on [18F]FDOPA PET/CT. Eur J Nucl Med Mol Imaging. 2022;49 (11):3787-3796. doi: 10.1007/s00259-022-05816-7. PubMed PMID:35567626 PubMed Central PMC9399031.
- Humbert, O, Bauckneht, M, Gal, J, Paquet, M, Chardin, D, Rener, D et al.. Prognostic value of immunotherapy-induced organ inflammation assessed on 18FDG PET in patients with metastatic non-small cell lung cancer. Eur J Nucl Med Mol Imaging. 2022;49 (11):3878-3891. doi: 10.1007/s00259-022-05788-8. PubMed PMID:35562529 PubMed Central PMC9399195.
- Chardin, D, Humbert, O, Bailleux, C, Burel-Vandenbos, F, Rigau, V, Pourcher, T et al.. Primal-dual for classification with rejection (PD-CR): a novel method for classification and feature selection-an application in metabolomics studies. BMC Bioinformatics. 2021;22 (1):594. doi: 10.1186/s12859-021-04478-w. PubMed PMID:34911437 PubMed Central PMC8672607.
- Humbert, O, Chardin, D. Dissociated Response in Metastatic Cancer: An Atypical Pattern Brought Into the Spotlight With Immunotherapy. Front Oncol. 2020;10 :566297. doi: 10.3389/fonc.2020.566297. PubMed PMID:33072599 PubMed Central PMC7531255.
- Chardin, D, Paquet, M, Schiappa, R, Darcourt, J, Bailleux, C, Poudenx, M et al.. Baseline metabolic tumor volume as a strong predictive and prognostic biomarker in patients with non-small cell lung cancer treated with PD1 inhibitors: a prospective study. J Immunother Cancer. 2020;8 (2):. doi: 10.1136/jitc-2020-000645. PubMed PMID:32709713 PubMed Central PMC7380842.
- Gal, J, Bailleux, C, Chardin, D, Pourcher, T, Gilhodes, J, Jing, L et al.. Comparison of unsupervised machine-learning methods to identify metabolomic signatures in patients with localized breast cancer. Comput Struct Biotechnol J. 2020;18 :1509-1524. doi: 10.1016/j.csbj.2020.05.021. PubMed PMID:32637048 PubMed Central PMC7327012.
- Hoog, C, Koulibaly, PM, Dejean, C, Desdoits, T, Humbert, O, Barranger, E et al.. Comparison of 3 γ-probes for simultaneous iodine-125-seed and technetium-99m breast cancer surgery: NEMA standard characterisation with extended processing. EJNMMI Phys. 2020;7 (1):37. doi: 10.1186/s40658-020-00299-7. PubMed PMID:32504305 PubMed Central PMC7275111.
- Le Goas, M, Paquet, M, Paquirissamy, A, Guglielmi, J, Compin, C, Thariat, J et al.. Improving 131I Radioiodine Therapy By Hybrid Polymer-Grafted Gold Nanoparticles. Int J Nanomedicine. 2019;14 :7933-7946. doi: 10.2147/IJN.S211496. PubMed PMID:31686819 PubMed Central PMC6777639.
- Martis, N, Levraut, M, Humbert, O, Lidove, O. Comment on: Muscle fluorodeoxyglucose uptake assessed by positron emission tomography-computed tomography as a biomarker of inflammatory myopathies disease activity. Rheumatology (Oxford). 2019;58 (12):2344-2345. doi: 10.1093/rheumatology/kez425. PubMed PMID:31608941 .
- Heeke, S, Benzaquen, J, Long-Mira, E, Audelan, B, Lespinet, V, Bordone, O et al.. In-house Implementation of Tumor Mutational Burden Testing to Predict Durable Clinical Benefit in Non-small Cell Lung Cancer and Melanoma Patients. Cancers (Basel). 2019;11 (9):. doi: 10.3390/cancers11091271. PubMed PMID:31470674 PubMed Central PMC6769455.
- Hafsi, M, Preveral, S, Hoog, C, Hérault, J, Perrier, GA, Lefèvre, CT et al.. RGD-functionalized magnetosomes are efficient tumor radioenhancers for X-rays and protons. Nanomedicine. 2020;23 :102084. doi: 10.1016/j.nano.2019.102084. PubMed PMID:31454552 .
- Decazes, P, Hapdey, S, Humbert, O, Marie-Cardine, A, Vera, P. Uptake of Pineal Gland Visible on 18F-DOPA PET/CT With Last Generation of SiPM PET/CT. Clin Nucl Med. 2020;45 (1):e67-e68. doi: 10.1097/RLU.0000000000002742. PubMed PMID:31348082 .
- Humbert, O, Bourg, V, Mondot, L, Gal, J, Bondiau, PY, Fontaine, D et al.. Correction to: 18F-DOPA PET/CT in brain tumors: impact on multidisciplinary brain tumor board decisions. Eur J Nucl Med Mol Imaging. 2019;46 (7):1581. doi: 10.1007/s00259-019-04321-8. PubMed PMID:30980100 .
- Grapin, M, Coutant, C, Riedinger, JM, Ladoire, S, Brunotte, F, Cochet, A et al.. Combination of breast imaging parameters obtained from 18F-FDG PET and CT scan can improve the prediction of breast-conserving surgery after neoadjuvant chemotherapy in luminal/HER2-negative breast cancer. Eur J Radiol. 2019;113 :81-88. doi: 10.1016/j.ejrad.2019.02.005. PubMed PMID:30927964 .
2011 - Award-winner at the Faculty of Medicine, Dijon, France (Prix de these).
iBV - Institut de Biologie Valrose
"Tour Pasteur"
Université Nice Sophia Antipolis
Faculté de médecine
28 Avenue de Valombrose
06189 Nice cedex 2